Data science is an emerging discipline which combines analysis, programming and business knowledge and uses new and advanced techniques and technologies to work with complex data. A group across Cabinet Office, Government Office for Science and ONS is exploring the potential of data science to improve policy making and operational delivery across a range of departments. Meanwhile, HMRC are using it to tackle non-compliance. In the first of our Data Science series, Jonathan Athow, John Lord and Claire Potter, from Knowledge Analysis and Intelligence at HMRC, explain how better use of data is changing the way the department works.
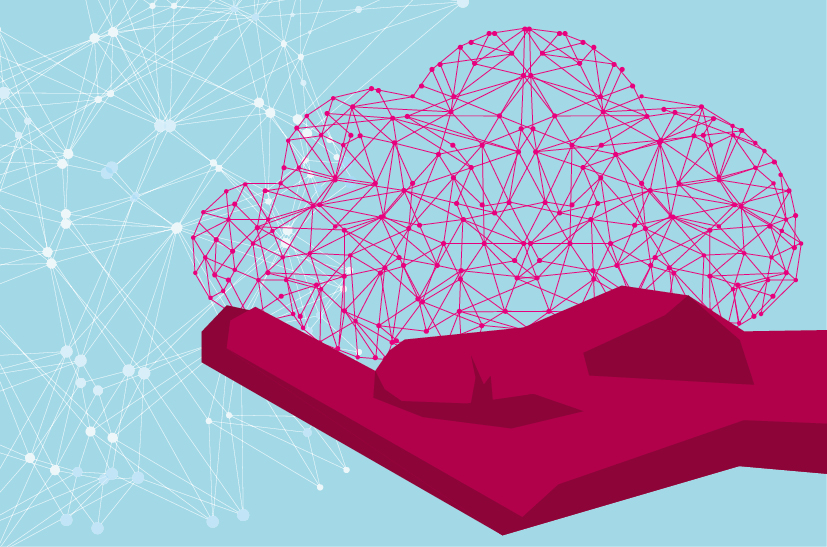
Analytics is a word on everyone’s lips and the Harvard Business Review carried an article titled ‘Data Scientist: The Sexiest Job of the 21st Century’. But what is analytics and who are the data scientists? And what does this all mean for HM Revenue and Customs and government more widely?
Analytics can be defined as identifying meaningful patterns in data. It is an approach that has become common place for many businesses, who use analytics to run or improve their operations. Online retailers use analytics to predict the goods and services you might be interested in by examining your past behaviour and personal characteristics and comparing these to how people with similar characteristics have behaved in the past. That understanding of past behaviour can be gained by analysing millions, or even billions, of previous transactions.
Predictive analytics
Analytics has a long history in some industries. In the financial sector, banks and other financial institutions have used analytics to undertake credit risking. Who should be given a loan and who should be denied one? Looking at your personal circumstances such as income, employment type and past credit history, can enable a financial institution to predict how risky a proposition you are, based on past experience with people in similar circumstances.
This approach is called ‘predictive analytics’ and is one of the main categories of analytics. This means using the patterns of past behaviour to predict behaviour in the future. There are other ways in which analytics can be used, from understanding customers to optimising a business process. Analytics itself uses a number of mathematical and statistical techniques to help sort through large amounts of data.
Data scientists
The term ‘data scientist’ is even more recent than analytics. It is the data scientist’s job to turn data into useable information, often doing the ‘heavy lifting’ with complex or even unstructured data that reveals new customer insights. The chief skills of a data scientist comprise being comfortable with both large data sets, and the IT systems and tools used to manipulate them. The data scientist is also able to apply the knowledge gained from the data to improve the way a business is run. The term is now expanding to cover some traditional areas of analysis and boundaries are becoming blurred.
In government, we have very few data scientists across departments and professions. They are usually found within the statistics and operational research professions who have the closest baseline skills needed for data science. However, the combination of statistics, maths, coding and business knowledge used alongside new tools, techniques and data sources is how data scientists in the private sector and academia are gaining new insight. The backgrounds of this wider community of data scientists is eclectic, from palaeontology to particle physics.
Analytics and HMRC
Having good analytics, with the right people and technology, is important for HM Revenue and Customs. The problem solving skills and approaches of analytics help the department understand how to deploy its resources most effectively. At a working level, analytics is used to help focus our compliance activities on those we think most likely to be non-compliant, be that making errors in their tax returns or deliberately trying to evade tax.
Using similar techniques to those used in credit scoring, we are able to build models to identify which taxpayers are likely to be understating their incomes. This can be used, alongside other information, to target our compliance interventions.
How predictive analytics works: decision trees
In HM Revenue and Customs we have used a number of techniques to develop analytics models. One technique we have found particularly useful is decision tree analysis, which presents operational colleagues with a diagram showing how characteristics affect the risk of non-compliance.
The following is a stylised example of how decision trees can be used to identify non-compliance in the tax system. The process works broadly as follows:
- We start by collecting information about whether taxpayers have been compliant or non-compliant in the past – the outcome.
- We add to this information we know about the customer from their tax return such as their income, age and occupation – the inputs.
- We use decision tree algorithms to sort customers based on their known characteristics (inputs) so we can derive the probability of the target outcome (the risk non-compliance).
- The resulting tree can be used to create rules which assign our customers to each final ‘leaf’ of the tree and so give the likelihood of each customer being non-compliant.
The decision tree below shows how the approach works. There is a population of 1,000 taxpayers of whom 30 per cent are non-compliant. They work in two sectors of the economy, either sector A or sector B. In addition, we know that some taxpayers are incorporated businesses while others are sole traders. We can use the relationship between their characteristics – that is the sector of the economy they work in or the nature of their business – and their likelihood of being non-compliant to help target which taxpayers we investigate.
Following through the decision tree, we find that people in sector B of the economy are more likely to be at risk of non-compliance than those in sector A. Further, among those in sector B of the economy, it is the incorporated businesses who are the most risky group of all. This information could be used to target our compliance efforts on the part of the population presenting greatest risk – doubling the chance of identifying the non-compliant taxpayers while needing to investigate less than half of the population.
This is a stylised example and characteristics discussed are not the real ones we use to identify the non-compliant. It does, however, illustrate the overall approach. In reality we are able to use a wide variety of data to help identify the categories of people who present most risk. As our data grows, so does our ability to better protect the Exchequer from tax evasion and other threats.
A variety of analytics techniques are currently used in HM Revenue and Customs, alongside other approaches, to help realise substantial sums for the Exchequer. Underpinning the new contract with the private sector to tackle error and fraud in the Tax Credit system are analytics models based on the ‘decision tree’ approach. We are expecting this measure to help bring in up to £1 billion in the next four years.
Elsewhere in the Department we are using analytics models to help tackle VAT evasion, where we estimate the improved targeting will bring in around £200 million a year in additional revenue. We are currently extending our modelling to include new populations such as Self Assessment taxpayers. The early indications here are promising with initial trials of the new model suggesting it will double the amount of revenue collected from each caseworker.
Giving a nudge
The world of analytics is constantly changing and new challenges and opportunities present themselves. HM Revenue and Customs wants to use data and analytics to shape more of its work. Can we find ways of nudging customers into changing their behaviour either to increase the chances of taxpayers being more compliant in the first place or acting in a way that saves the Department money?
The answer is yes. As a department we have already successfully tried a proof of concept aimed at those who could switch to filing their Self Assessment return online rather than more expensive paper filing. We were able to identify some of those most likely to change and, working with customer insight experts, tailor communications to those taxpayers to encourage them to move from paper to online filing. Evaluation showed a shift from paper to online filing of around 10 per cent.
Joining the dots
Analytics is, however, only one part of the picture. In the case of the Self Assessment proof of concept, it needed to be combined with accurate and up-to-date information about our customers. Analytics rests on the foundation of data and the IT systems needed to make the most of that data. The new digital services that HMRC is developing will be a significant step forward in enhancing the richness and timeliness of the data available for analytics.
As a Department, we must also use tools such as analytics carefully. People trust HM Revenue and Customs with personal data and rightly expect us to use that data appropriately. Our use of data and analytics therefore needs to be properly considered and proportionate.
The future for analytics in HM Revenue and Customs is bright. It is a very useful tool to help us tackle tax non-compliance, improve customer service and reduce costs. Analytics will provide us with even greater benefits as we continue to build our capabilities in terms of people, skills, technology and data.
5 comments
Comment by Steven Finlay posted on
Nice article. Good to see government using modern analytical tools to benefit of the public good.
Comment by Alec Tasker posted on
Not sure that using the banking sector is a good example. Using 'analytics' to decide who to loan to crashed the world economy.
There is a great deal of quackery in this area. If you look at a large data set for long enough, you can see all sorts of patterns that don't actually mean anything. The trick is to differentiate correlation from causation.
Comment by Shaun Morris posted on
All very well in theory and perhaps in HMRC there is lots of good quality data available. However, I suspect in many other parts of the Civil Service (my own part included) the data required to inform key decisions is often either not collected at all, or is not subject to compliance checks resulting in unreliable datasets.
Comment by Endlessly curious posted on
Really interesting - thanks. You can read the HBR article here: https://hbr.org/2012/10/data-scientist-the-sexiest-job-of-the-21st-century/
Comment by Steven Naylor, Scientist, Health and Safety Laboratory posted on
The Health and Safety Laboratory, the Health and Safety Executive’s scientific arm, is currently looking at how predictive analytic techniques can be used for benefit in health and safety, for example, to help asset intensive, major hazard organisations predict the occurrence of operation critical events such as key asset failures. Operators in the utilities, manufacturing and oil/gas sectors in particular, are becoming increasingly aware that predictive analytic approaches can help them shift their asset management systems away from the traditional reactive (scheduled, break-fix) maintenance type regime, and more towards a proactive (condition-based, preventive) maintenance type regime, i.e. where major outages are avoided in the first place rather than fixed after they happen. This area of predictive analytics application is being explored in a blog hosted by the US’s National Institute of Occupational Safety and Health for those interested in such areas of application. Highlights that predictive analytic approaches are now being used to very good effect in science, technology and engineering circles also. http://blogs.cdc.gov/niosh-science-blog/2014/10/02/pa/